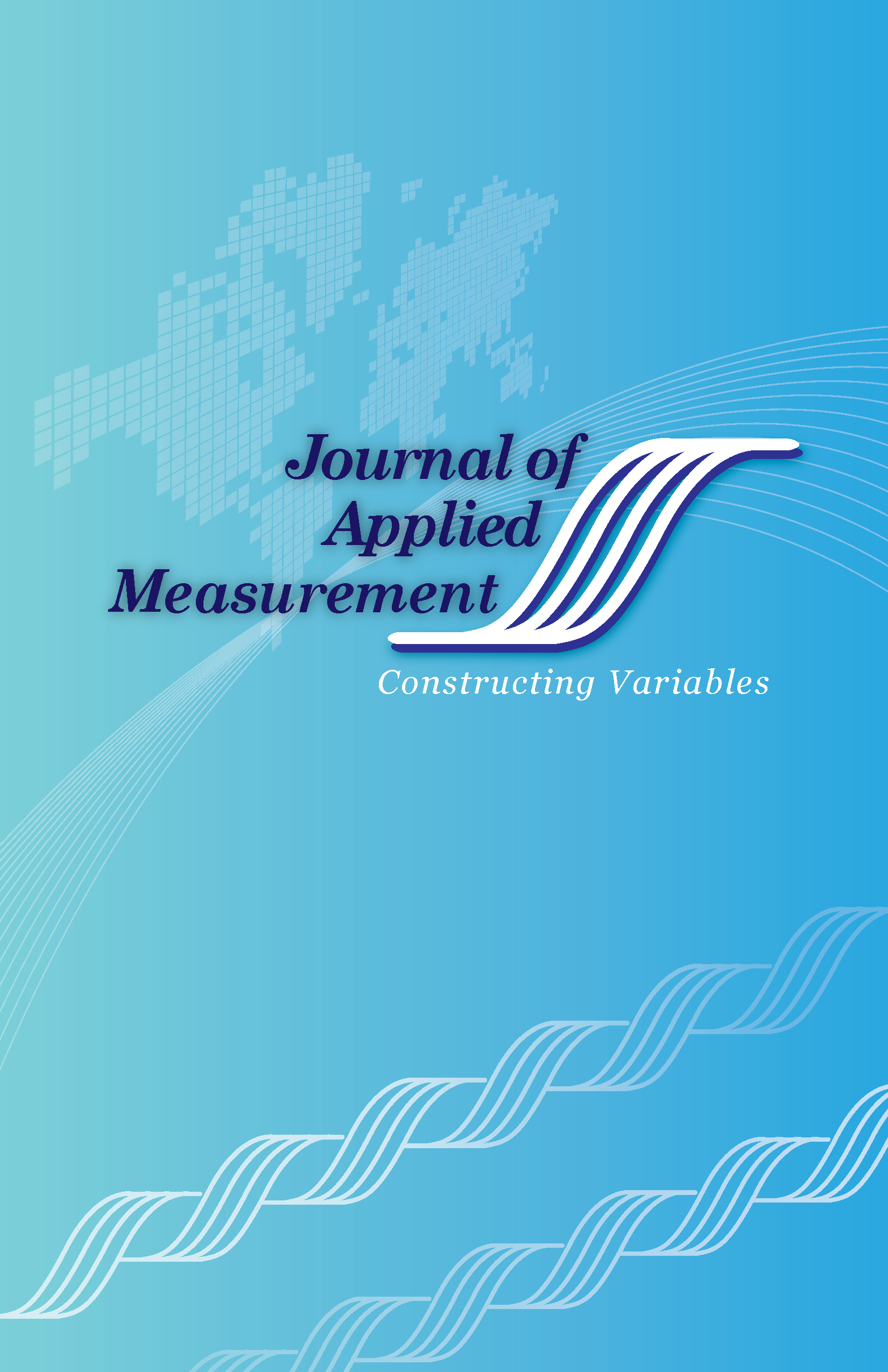
Journal of Applied Measurement
A publication of the Department of Educational Psychology and Counseling
National Taiwan Normal University
Journal of Applied Measurement Abstracts
Volume 23, Issue 1/2 (2022)
Special Issue on Unfolding Models
A Historical Perspective on Unfolding Models
George Engelhard, Jr.
Ye Yuan
The University of Georgia
The purpose of this study is to provide a brief history of unfolding item response theory models that includes a discussion of the detailed principles that undergird different models. The concept of an unfolding response process can be traced back more than 90 years to Thurstone. Thurstone distinguished between increasing-probability (cumulative) and maximum-probability (unfolding) scale types in the construction of attitude scales. A Web of Science search was conducted to identify trends and major milestones in research on unfolding models. Several key unfolding models for dichotomous responses are described and compared using a set of basic principles that define these models. A taxonomy for dichotomous unfolding models is described based on the principles that distinguish the models. Finally, suggestions for future research on unfolding models are presented.
Keywords: Unfolding models, item response theory, dominance models
Citation:
Engelhard, G., & Yuan, Y. (2022). A Historical Perspective on Unfolding Models.
Journal of Applied Measurement, 23(1/2), 1–18.
Accuracy and Precision of Bayesian Multigroup Estimation for the Generalized Hyperbolic Cosine Ideal Point Item Response Theory Model
Sean Joo
University of Kansas
Philseok Lee
George Mason University
Stephen Stark
University of South Florida
In educational and psychological assessment, there is often a great interest in comparing parameters estimated in different examinee groups or subpopulations. This issue is typically pursued using either a separate calibration and linking strategy or through simultaneous (concurrent) calibration of responses of multiple groups using anchor (common) items to set a base metric. To date, multigroup estimation methods have been developed for many dominance item response theory (IRT) models, but there has been relatively little research with ideal point models that may be useful for noncognitive measurement. To address this research gap, we developed a multigroup estimation algorithm for the generalized hyperbolic cosine model (GHCM) under a Bayesian framework, and we conducted a Monte Carlo simulation study to evaluate its efficacy with varying levels of sample size, test length, data type, and subpopulation trait distribution. We found this algorithm accurately estimated item and latent trait parameters across multiple groups with minimal bias. The implications of these findings and practical recommendations involving the ideal point IRT model are further discussed.
Keywords: generalized hyperbolic cosine model, item response theory, Markov chain Monte Carlo, multigroup analysis, ideal point
Citation:
Joo, S., Lee, P., & Stark, S. (2022). Accuracy and precision of Bayesian multigroup estimation for the
generalized hyperbolic cosine ideal point item response theory model.
Journal of Applied Measurement, 23(1/2), 19–36.
Fitting Hyperbolic Cosine Unfolding Models Using the Stan Language
Jue Wang
University of Science and Technology of China
Trenton Combs
University of Miami
Cengiz Zopluoglu
University of Oregon
Unfolding models, also referred to as ideal point models, are typically used to measure attitudes, preferences, and personality. Available computer packages for fitting unfolding models are very limited compared with a wide selection of computer programs for fitting other item response theory models. This impedes the methodological development of unfolding models and their applications in educational and psychological areas. This study aims to demonstrate fitting unfolding models using Stan, a Bayesian statistical software program based on the Hamiltonian Monte Carlo algorithm. We provide an accessible tool for fitting unfolding models, which will help researchers and practitioners to conduct research studies with unfolding models. We design the Stan program based on a hyperbolic cosine model (HCM; Andrich, 1993) within the Bayesian framework and provide illustrations through empirical and simulation examples. The Stan program showed efficient convergence for HCM analysis with accurate and reliable parameter estimates, which has great potential for unidimensional unfolding analyses.
Keywords: Unfolding models, Hyperbolic cosine model, Stan program, Bayesian estimation, Hamiltonian Monte Carlo algorithm
Citation:
Wang, J., Combs, T., & Zopluoglu, C. (2022). Fitting hyperbolic cosine unfolding models using the Stan
language.
Journal of Applied Measurement, 23(1/2), 37–60.
A Preference Model Based on an Unfolding Response Process: A law of Comparative Preference
David Andrich
The University of Western Australia
Thurstone’s Law of Comparative Judgment (LCJ) is intended to provide a comparison between the values of a pair of objects on a measurement scale, independent of any preference of the person making the comparison. The basis for the mathematical expression of the LCJ is a latent, cumulative, discriminal process when a person engages with each object, a process characterized by an increasing-probability model. It can be shown that in the mathematical expression of the LCJ based on this model, a formalized person parameter is eliminated experimentally reflecting the intention that the comparison is an impersonal judgment. This paper shows the construction of the comparison between a pair of objects where, in addition to the scale values of the pair of objects, a formalized person parameter is an integral part of the comparison. This has been termed the Law of Comparative Preference (LCP) which reflects that the comparison is a personal preference. This paper shows that the basis for the mathematical expression of the LCP is a latent, unfolding, discriminal process when a person engages with each object, a process that can be characterized by a maximum-probability model. It is shown that in the mathematical expression of the LCP, the person parameter cannot be eliminated experimentally. Suggestions for areas of application of the model are provided together with some benefits and challenges.
Keywords: Unfolding response processes, law of comparative judgment, law of comparative preference
Citation:
Andrich, D. (2022). A preference model based on an unfolding response process: A law of comparative
preference.
Journal of Applied Measurement, 23(1/2), 61–73.
Commentary on the Special Issue on Unfolding Models
Wendy J. Post
Department of Child and Family Welfare,
University of Groningen, Groningen,
The Netherlands
The papers of this special issue address interesting and important issues with respect to unfolding models.
The special issue started with a paper on historical perspectives, followed by a paper about multigroup
estimation for the generalized hyperbolic cosine model (GHCM), a paper about fitting the hyperbolic cosine
model (HCM) using STAN language, and ended with a paper about a preference model based on an unfolding
process. With the focus of two papers on Bayesian approach and on (G)HC models in the latter three papers,
it is worth mentioning that this special issue is covering only a part of the wide range of research in
unfolding models. Nevertheless, they provide promising input for further unfolding research.
I will start with my commentary for each paper separately, followed by discussing various topics that
sparked my interest during reading these papers. It concerns the application of unfolding models in
empirical research together with the motivating choice between unfolding and dominance models, and person
fit issues.
Citation:
Post, W. (2022). Commentary on the special issue on unfolding models.
Journal of Applied Measurement, 23(1/2), 74–79.